Project by: Cynthia Hua, Devin Zhang, Gaurav Patekar
In the participatory game project, We created a web-game, Cars in Space!, to provide a fun and accessible educational experience on why and how to choose greener cars. We used the Environmental Protection Agency’s Fuel Economy Data which shows how fuel-efficient cars can produce drastically lower CO2 emissions, while also saving the driver money due to reduced gas costs. Link to the sketch: https://datastudio2020.datatherapy.org/2020/04/22/cars-in-space-a-data-game/
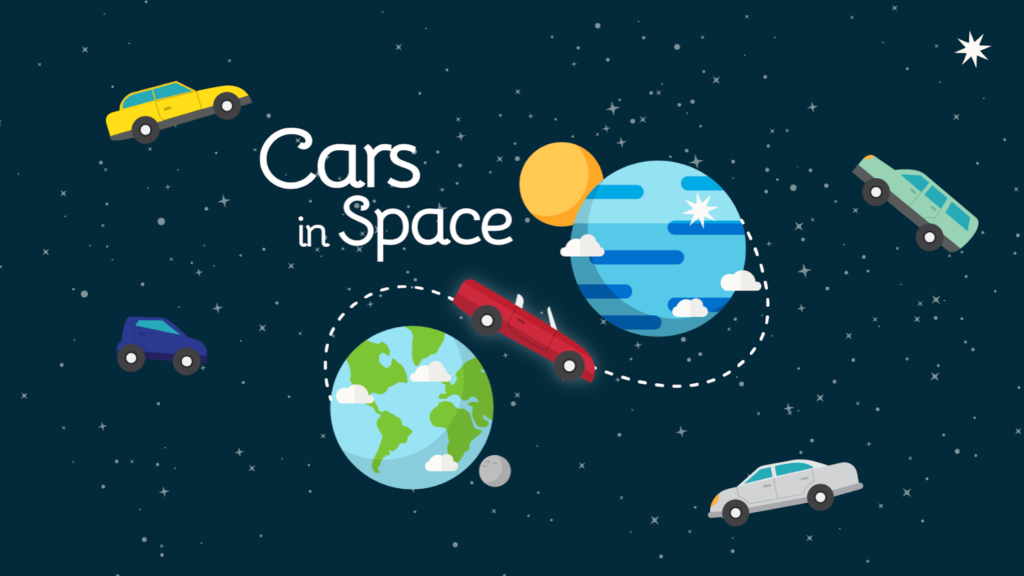
As the game was well fleshed out in terms of the game mechanics and visual style, we chose to keep it same as before. We shortened the original slide deck to include only the game screens and info on the game play to be shared with prospective users.
Audience:
Our target audience is young car buyers aged 18 – 40. This might include a college student buying a first car, a young professional buying a car for work or a parent buying a family car. We are interested in young buyers because we believe we can impact their car buying decisions over a lifetime by educating them early and because young people have the most cause to be invested in a cleaner environment.
Goals:
Through a logic modeling exercise we mapped out activities, the output of the game as well as the desired short, mid and long term impact.
Activities | Output | Short Term Impact | Mid Term Impact | Long Term Impact |
Play car riding game | Get to do a fun activity | Feel good | Tell friends about the game | |
Choose cars | Learn about cars and pollution | Awareness of car pollution and car types | ||
Read popups about pollution | Get to know more about emissions, reiterated in the printable car buying guide | Tell others about car pollution | Share learnings with friends and family buying cars | Internalize the learning about car types, sizes and pollution |
Read popups about upgrades | Get to know various car names, reiterated in the printable car buying guide. | Sense of wokeness | ||
Get a printable car buying guide | Get to know how cars compare to each other | Apply learnings to car buying decisions | Apply learnings to car buying decisions |
Based on the impact we wanted to test, we chose to go with a semi-structured interview method as it would allow us to collect qualitative data that we could use to improve the game.
We spoke to 5 prospective users selected as per the audience criteria listed above (age 18-40, considering buying their first car). Since the game is not interactive at the moment, we felt it would better to give a brief run-through of the game to the users over a call, answer any doubts they have about the game and then proceed with the interview questions. We had a series of questions for the interview covering many of the impacts listed above. Each interview lasted about 15-40 minutes. Link to the questions and the interview data: https://docs.google.com/spreadsheets/d/1S_9dh0DbCIFlCNdDzhwzjlPcpD7DC_wTMml8Pa2RQlk/edit?usp=sharing
Feedback from the interviews:
We found that most of our users rely on online sources of information for car research along with personal experience in some cases. None of the users directly referred to the car fuel efficiency data from EPA.
Prior to the interview, the most important factors for the users were the price of the car, fuel costs along with environmental impact. When we asked the same question post-interview, the answers still remained similar, price and fuel costs were still the important factors but there was an increased awareness of the environmental impact.
While 3 out of 5 users said they would click on this game 2 users did not want to do that if the game appeared as an advertisement, all users were willing to play the game for 2 – 10 minutes, in terms of game enjoyment, for the majority of the users it was conditional on how the final game would look and feel and the complexity of the gameplay. All the users were able to understand the game and gameplay after the initial run-through.
We got valuable feedback about if the users found the information useful and if it would be helpful to them in their car buying decision. The majority of the users found the information about CO2 emission new and educational. There was feedback from one user about the information being too simple. One of the users mentioned how they don’t really know what to make of the numbers as they seem to abstract to them and suggested contextualizing the numbers through the number of trees burnt or breathing problems developed through deterioration in air quality.
While some users were able to relate the cars in the game to real-life cars, some users found it difficult because of the cartoonish style of the graphics and mentioned how the style gives them the impression that the game is meant for a younger audience.
Users in general found the popups in the game informative, some of the users suggested making the popups shorter or make the key piece of imfromation stand out better as currently there is a lot of text in the game.
Users saw value in the car buying guide at the end of the game. Some of the users said they would prefer an image they can share on social media instead of printing the guide.
In terms of additional inputs, users asked for additional levels of complexity in the game to keep them engaged, there were recommendations for periodically updating the game with data for newly released cars, including information about the longevity of cars, carbon emissions from the car manufacturing process and the impact of using alternate fuels